The Relationship Between LLMs and Generative AI: A Deep Dive into the Future of TechnologyHello world!
Overview
In recent years, the rise of Generative AI has captured the imagination of the tech world, promising to revolutionize industries ranging from entertainment and marketing to healthcare and finance. At the heart of this wave of innovation are Large Language Models (LLMs), which power many of the generative AI applications we see today. But what exactly is the relationship between these two concepts, and how do they work together to create AI systems that can understand, generate, and even transform human language?
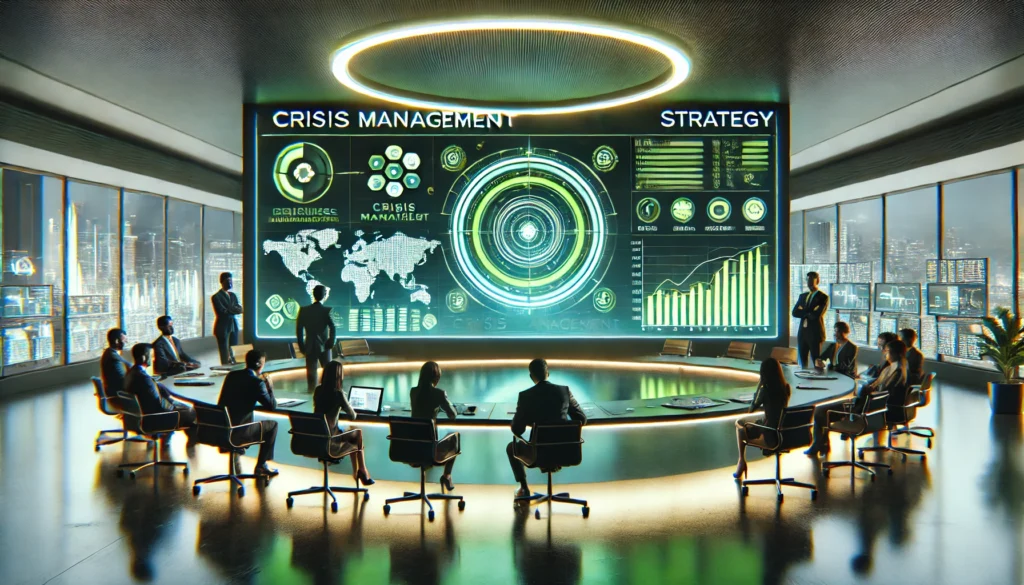
What is Generative AI?
Generative AI refers to a class of algorithms that can create new content based on patterns and data it has learned from. Unlike traditional AI, which may focus on classifying or recognizing data, generative AI models are designed to produce something new — whether that be text, images, music, or even code. These models are trained on vast datasets to understand the underlying structure of the information they are presented with, allowing them to generate realistic, coherent outputs that mimic the data they learned from.
Generative AI has gained significant traction in recent years with the success of tools like OpenAI’s GPT (Generative Pretrained Transformer), DALL·E, and MidJourney, which can generate human-like text, images, and other forms of content. These tools rely on the principles of generative AI to produce outputs that can be astonishingly accurate and creative.
What are LLMs?
Large Language Models (LLMs) are a subset of generative AI that specialize in understanding and generating human language. These models, like GPT-3, GPT-4, and others, are trained on massive datasets consisting of books, websites, and other text-based content. The sheer scale of the data they are trained on allows LLMs to learn complex patterns in language, such as grammar, sentence structure, contextual meaning, and even nuances in tone and style.
At their core, LLMs are deep learning models that use the transformer architecture, a type of neural network that excels at handling sequences of data, like text. The transformer model enables LLMs to generate coherent, contextually aware sentences and paragraphs, making them incredibly useful for a wide range of applications, from content creation to customer service chatbots and beyond.
How LLMs Power Generative AI
LLMs are the driving force behind many of the most impressive generative AI systems we use today. By leveraging their understanding of language, LLMs can create high-quality text, answer questions, summarize articles, and even engage in detailed conversations. This ability to generate human-like language is what makes tools like ChatGPT and Bard so revolutionary.
- Text Generation: LLMs can generate written content across various genres, from poetry and stories to technical articles and business reports. By analyzing the patterns in the input text, LLMs can predict the next word or phrase, eventually producing entire paragraphs that are logically consistent and contextually relevant.
- Personalization: One of the key features of LLM-powered generative AI is its ability to tailor content to specific audiences or individuals. Through techniques like fine-tuning and transfer learning, LLMs can be adjusted to produce content in specific domains, such as legal, medical, or financial language, allowing for highly specialized outputs.
- Multimodal Capabilities: LLMs aren’t just limited to text; they can also be combined with other forms of AI, such as image generation models like DALL·E, to create multimodal outputs. This integration of different AI models broadens the scope of what generative AI can do, enabling the creation of content that includes both text and visuals, like infographics, illustrations, and even deepfake videos.
- Interactive Systems: By embedding LLMs into conversational AI systems, businesses can create chatbots or virtual assistants that can converse naturally with customers. These systems can handle complex inquiries, offer personalized responses, and even simulate human-like interactions.
Real-World Applications of LLMs in Generative AI
The relationship between LLMs and generative AI has led to the development of powerful tools with real-world applications:
- Content Creation: Writers, marketers, and journalists use LLMs to generate articles, social media posts, blog content, and product descriptions. With the ability to produce text quickly and efficiently, LLMs are reshaping the content creation process.
- Entertainment and Media: LLMs have found a place in scriptwriting, songwriting, and video game development, where they assist in creating engaging narratives and dialogue for films, games, and other forms of media.
- Code Generation: Platforms like GitHub Copilot use LLMs to assist developers by generating code snippets, debugging, and suggesting improvements based on the context of the code being written.
- Customer Support: AI-powered chatbots that use LLMs can handle customer queries, providing quick and accurate responses. These systems can learn over time, offering better customer service experiences.
- Healthcare: LLMs are being used to analyze and generate medical texts, such as patient records, research papers, and diagnostic reports. Their ability to process vast amounts of information allows healthcare professionals to receive valuable insights and recommendations.
The Future of LLMs and Generative AI
The potential for LLMs and generative AI to change the way we interact with technology is limitless. As these models continue to evolve, we can expect even more sophisticated capabilities, such as:
- Greater Creativity: As generative AI becomes more advanced, we may see AI systems that can not only replicate existing styles but also create entirely new art forms, music genres, and writing techniques.
- Improved Personalization: With more tailored and context-aware LLMs, generative AI will be able to provide highly personalized content, from customized learning experiences to hyper-specific recommendations.
- Cross-Domain Capabilities: The future could see AI models that can seamlessly integrate knowledge from different fields, allowing for more holistic solutions to complex problems in areas like healthcare, business strategy, and scientific research.
Ethical Considerations
While the relationship between LLMs and generative AI is promising, it also raises important ethical concerns. These include the potential for misuse in generating misleading or harmful content, biases present in the models due to skewed training data, and the impact on jobs traditionally held by humans, such as content writing or customer service.
As these technologies advance, it will be crucial for developers, regulators, and society as a whole to address these issues, ensuring that AI is used responsibly and for the betterment of society.
Conclusion
The relationship between LLMs and generative AI is a powerful one, driving the next generation of technology that is transforming how we interact with machines and the world around us. From enhancing creativity to improving efficiency across various industries, the possibilities are vast. However, as we continue to push the boundaries of what these models can do, it is essential to remain vigilant about their potential consequences and ensure that AI develops in a way that benefits everyone. The future of LLMs and generative AI is bright, but it must be guided by careful consideration and responsible development.
Other Posts
Let's Drive Your Business Forward
Ready to take your business to the next level? Let Witty Solutions guide you with expert strategies and innovative solutions. Let’s drive success together!